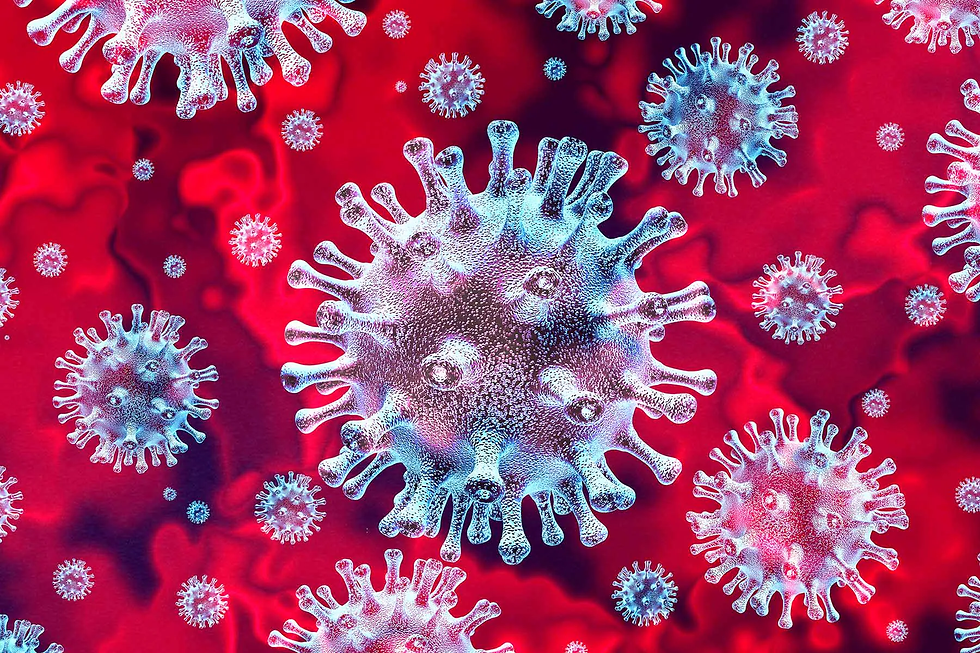
Data
Methodology
Filtering Data:
​
After obtaining data from the City of Chicago Data Portal, we filtered through the CSV files using Excel to minimize the dataset into the specific data we needed. We began by filtering the crime data using the Illinois Uniform Crime Reporting Codes. This data included all crime types and reported incidents from 2001 to 2021, which was far more data than necessary for our study. To organize this file more efficiently, we filtered by crime type according to assigned crime codes. We specified domestic battery including domestic battery simple and aggravated domestic battery with and without weapons; the respective crime reporting codes being: 496, 497, 498, and 486. We then filtered by year, to compare domestic abuse rates one year before lockdown, from March 21, 2019 to March 21, 2020, against abuse rates after the lockdown order went into effect from March 22, 2020, to March 22, 2021. Unfortunately, we were unable to access population data for both 2019 and 2020, and instead used data from 2019 by zip codes. Before continuing our analysis, we obtained the remainder of our data, all being from the City of Chicago Data Portal.
Joins:
​
After uploading the shapefiles and data to ArcGIS Pro, we started by joining our 2019 population count data to our zip codes shapefile by zip code. From here, we displayed the XY data from the filtered domestic battery dataset for both years of study; March 21, 2019, to March 21, 2020 and March 22nd, 2020 to March 22nd, 2021. We performed a spatial join using these points to the zip code shapefile containing the population data. We then conducted a percent change analysis to explore the increase or decrease in domestic battery cases between pre- and during-COVID-19 years. After joining the March 2019/20 crime data to March 2020/21 crime data, we were then able to calculate the percent change (using the percent change tool) for domestic abuse rates before and after lockdown orders went into effect.
​
Statistical Models:
​
For statistical analysis, we moved to the community area scale which represents each neighborhood of Chicago. A shapefile containing the names of each community and demographic data assigned to the same area was obtained from the City of Chicago Data Portal. In ArcPro, the table containing the demographic data was joined to the shapefile. The shapefile was then used to create two different maps; joined in the same manner as before, one showing pre-COVID-19 domestic violence cases and one showing instances during-COVID-19. These were used for statistical analyses exploring the relationship between select demographic factors and domestic violence.
Each individual year of data and the change of domestic battery rates across both years of study received the same analytical analysis. An exploratory regression was run on the data set to determine which demographic variables were the most significant with regard to count instances of domestic battery. The model was run again with the most significant variables to determine further significance. Next, a generalized linear regression (GLR) was performed using count instances as the dependent variable and the variables selected during the exploratory regression as the independents. Lastly, a geographically weighted regression (GWR) was performed on the data using the same variables as the GLR in order to determine if geography plays a significant role in this study.