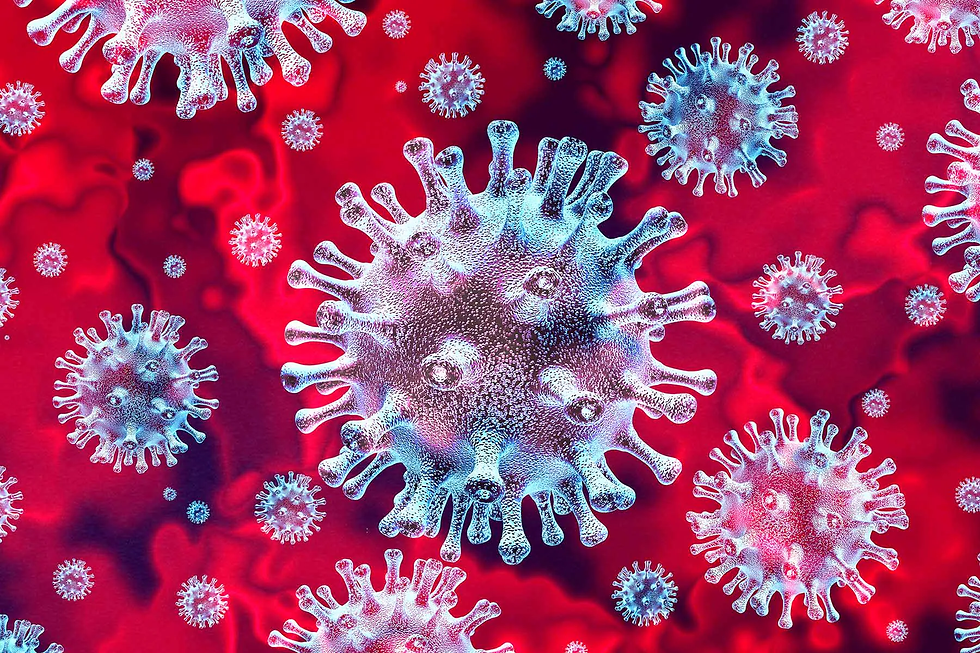
Limitations
Error can occur throughout a GIS analysis at various points, and has the potential to combine with other errors introduced to the GIS in unpredictable ways and significantly disrupt the analysis (Unwin, 1995). What follows are some errors we uncovered in our analysis:
​
While collecting our data, we found a lack of population and demographic data available from the US Census Bureau at the spatial scale we required, therefore we obtained all our data from the City of Chicago Data Portal. However, this had some limitations. The population dataset provided was data from 2018 and 2019. We used the 2019 data as it was more recent, but our analysis was built on a very specific time frame from the year leading up to the pandemic to the end of the first year of the pandemic, meaning that this data was not ideal. To accurately map the change in rate of domestic abuse before and during the pandemic, population data from March 21st, 2019 to March 21st, 2021 would be needed. The demographic data was updated less recently than this; being from 2014. Therefore, because the years of both population counts and demographic data do not match the year of recorded domestic abuse incidents, it is important to consider this as a source of error in this analysis.
​
Another source of error present in this analysis is what some geographers refer to as, ‘one of the most stubborn problems in spatial analysis’: the modifiable areal unit problem (Wong, 2009). Within geospatial analyses, results are contingent on the spatial unit selected for analysis. However, spatial units used are arbitrary and modifiable, subject to the whims of the governmental agencies that create them. This is the modifiable unit areal problem (MAUP) (Green & Flowerdew, 1996). Because there are no ‘natural’ geographies when working with socio-economic data as our analysis dealt with, zip codes and community areas are used as artificial spatial units with questionable results. Using different spatial aggregation schemes, statistical relations and patterns observed in the same data can differ significantly (Green & Flowerdew, 1996).
​
As exploratory regression was used to determine which predictors of domestic violence to use in subsequent models, while considered a valuable statistical method, it is important to note its controversy as a statistical model as well (Braun & Oswald, 2011). As “no clear best solution” exists for assessing the importance of predictors, it is wise to consider multiple approaches before conclusively deciding which predictors contribute the greatest explanation of variance in criterion (Braun & Oswald, 2011, p. 337). Furthermore, to avoid inferential mistakes, it is important that scholars and researchers view all results carefully before making interpretations. This is crucial as the predictors chosen in this step will be used in the GLR and GWR for further analysis and form the basis for the conclusions we draw from the data. A sensitivity analysis would have been a useful addition here to the change in model output when input variables are manipulated (Gorris & Yoe, 2014). This systematic investigation would help us to understand how much error the model produces for each change in inputs. Thus, without this crucial step we lack an in-depth understanding of the relationship between model inputs and outputs (Gorris & Yoe, 2014).
Finally, as the GWR model yielded fairly low explanatory power of the demographic variables to explain rates of domestic violence in Chicago, for further research to improve, additional variables are necessary. It is likely that more variables may contribute to an increase in model explanatory power (McLay, 2021). As various historical, political, social, a and cultural factors contribute to the vulnerability of victims, this may include information such as history of family violence, anti-social behaviour, and antecedents of prejudice and political conservatism (in the context of violence against women) (Canto, Vallejo-Martín, Perles, & Martín, 2020).